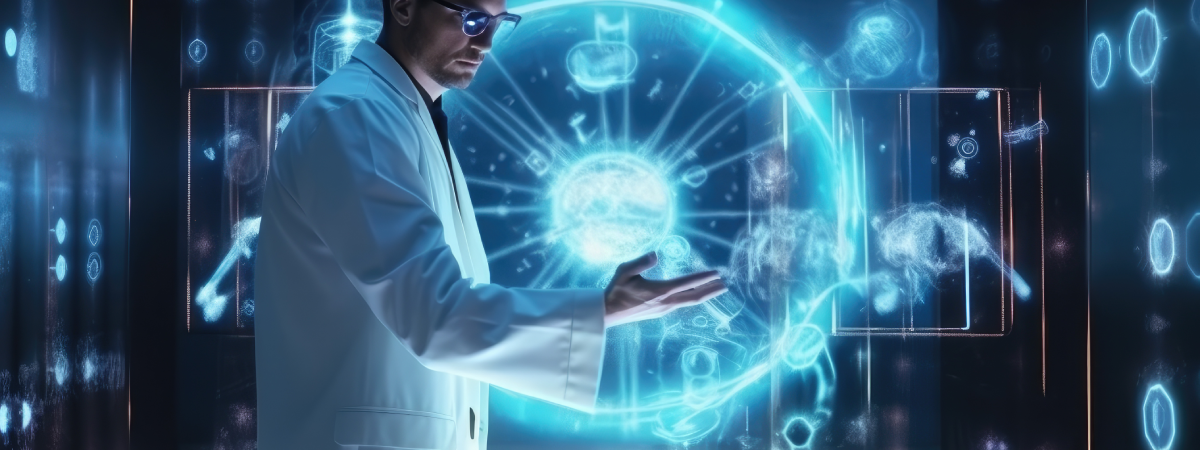
Holistic data quality and accessibility are foundational to effective care management and coordination, particularly for organizations such as IPAs, ACOs, and MSOs that prioritize value-based care. However, clinical data often suffers from inconsistencies, largely stemming from disparate data sources and poorly formatted or unstructured documentation. This blog explores common clinical data quality issues and how Generative AI can revolutionize this landscape.
Challenges with Clinical Data Quality
Value-Based Care prioritizes rewarding healthcare providers for improving the health outcomes of patients rather than the volume of services rendered. Through value-based contracting, healthcare plans and payers incentivize providers based on metrics tied to quality, financial outcomes, and patient satisfaction. These metrics often follow established standards like the Healthcare Effectiveness Data and Information Set (HEDIS) by NCQA or custom measures defined by payer organizations.
Challenges with Clinical Data Quality
1. Lack of Standardization in EHR Systems
While Electronic Health Record (EHR) systems boast nearly 100% adoption across various clinical settings (inpatient, outpatient, urgent care, etc.), their documentation methods vary significantly. Each practice employs its own methodology for recording patient chart data, leading to:
- Data Fragmentation:
Encounter records, ideally containing visit-related details like conditions, diagnoses, and lab tests, often become cluttered with unrelated patient communications. - Interoperability Issues:
Standardized data exchange between EHRs becomes challenging, making it hard for users to interpret and act on critical information.
2. Poor Formatting of Unstructured Data
Patient data encompasses both structured and unstructured information, with the latter including notes, discharge summaries, and other free-text documents. When transmitted across systems in HL7 messages, the original formatting is often disrupted, resulting in:
- Readability Issues:
Missing line breaks, inconsistent spacing, and jumbled information reduce the utility of these documents. - Impact on Care Coordination:
Case managers and providers struggle to sift through disorganized data, delaying interventions and increasing administrative burden.
Generative AI as a Solution
Generative AI, powered by large language models (LLMs), offers innovative solutions to the above challenges. By leveraging natural language processing (NLP) and machine learning capabilities, LLMs can process, structure, and enhance clinical data at scale.
Use Case 1: Structuring Visit Information
Identifying and classifying visit-specific information is a labor-intensive, repetitive process when done manually. With LLMs, this task becomes automated:
- Prompt Engineering:
A well-crafted instruction can guide the LLM to parse visit-related details (e.g., diagnoses, lab results) and classify them appropriately. - Accuracy and Speed:
The model can rapidly process plain-text data, minimizing human error and saving significant labor hours.
Use Case 2: Reformatting Documents for Readability
Reformatting unstructured documents manually is time-consuming. Generative AI can quickly improve document readability by addressing:
- Line Breaks and Spacing:
Restoring proper formatting to ensure logical information flow. - Context Preservation:
Retaining the integrity of the original document’s meaning while making it easier to read.
By automating these processes, Generative AI reduces administrative burden, enhances data quality, and accelerates patient care coordination.
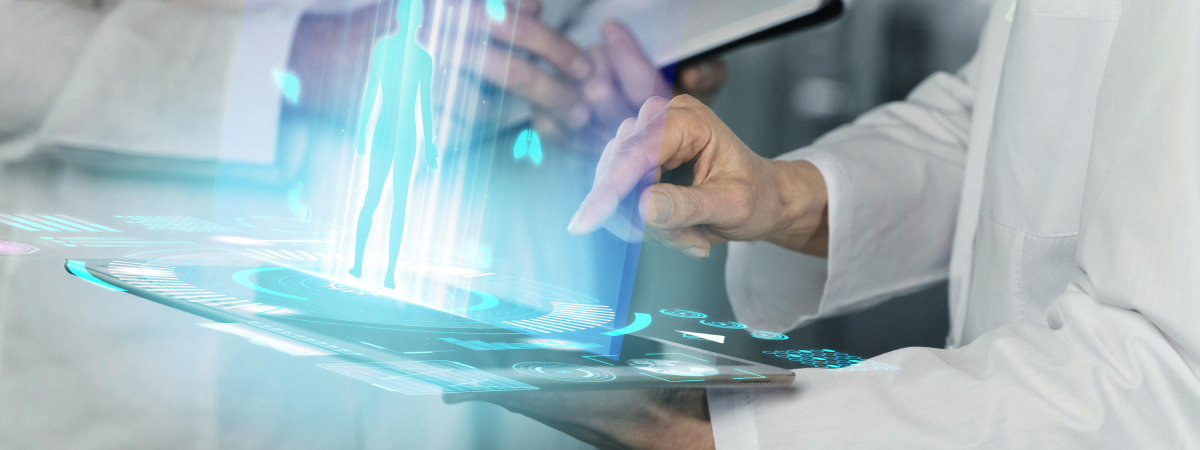
Challenges with LLM Implementation
While LLMs offer remarkable capabilities, their success hinges on effective prompt engineering. Some limitations to consider include:
- Contextual Understanding:
LLMs lack inherent knowledge and rely entirely on the prompts provided. Poorly worded instructions can lead to inconsistent results. - Edge Cases:
The model may require additional guidance for handling anomalies or unexpected data patterns.
To mitigate these issues, healthcare organizations must invest in crafting precise prompts and establishing contingency workflows for outlier scenarios.
Transforming Healthcare with Generative AI
The application of Generative AI to clinical data quality improvement presents enormous opportunities for the healthcare system. Key benefits include:
Timely Interventions:
Case managers and care coordinators can act faster, improving patient outcomes and satisfaction.
Reduced Provider Burden:
Automated data processing minimizes manual work, allowing providers to focus on care delivery.
Cost Efficiency:
Improved data quality and streamlined workflows help reduce administrative costs and meet performance objectives for value-based care.
By adopting Generative AI, organizations such as IPAs and ACOs can enhance care coordination, achieve at-risk value-based care goals, and ultimately deliver better patient outcomes.